AI deep technology has emerged as one of the most transformative forces in modern times, reshaping industries, businesses, and daily lives. Artificial intelligence, specifically deep learning, has gained significant traction due to its ability to process vast amounts of data and recognize patterns with incredible accuracy. This technology is not just a buzzword; it represents a paradigm shift in how machines learn and interact with the world.
AI deep learning is revolutionizing sectors such as healthcare, finance, transportation, and entertainment. By mimicking the human brain's neural networks, AI deep systems can perform tasks that were once considered exclusive to human intelligence. These systems are capable of understanding natural language, recognizing images, and even making predictions based on complex datasets.
As we delve deeper into the world of AI deep, it becomes evident that this technology is not just about automating processes but also about enhancing human capabilities. Whether it's improving medical diagnoses, optimizing supply chains, or creating personalized user experiences, AI deep is paving the way for a smarter, more connected future.
Read also:Madelyn Cline Height In Feet A Comprehensive Guide
What is AI Deep Learning?
AI deep learning is a subset of machine learning that uses artificial neural networks to process data in layers. These networks are designed to mimic the human brain's structure, enabling them to learn from vast amounts of data and improve over time. Unlike traditional machine learning algorithms, deep learning models can automatically extract features from raw data, making them highly effective for tasks such as image recognition, speech processing, and natural language understanding.
How Does AI Deep Learning Work?
AI deep learning operates through a series of layers, each responsible for extracting specific features from the input data. The first layers typically handle basic features such as edges or colors, while subsequent layers focus on more complex patterns. This hierarchical approach allows deep learning models to achieve remarkable accuracy in tasks that require nuanced understanding.
- Input Layer: Receives raw data
- Hidden Layers: Process data and extract features
- Output Layer: Provides the final result
Applications of AI Deep Learning
AI deep learning has found applications across various industries, driving innovation and efficiency. Below are some of the key areas where this technology is making a significant impact:
Healthcare
In healthcare, AI deep learning is being used to improve diagnostic accuracy, predict disease progression, and personalize treatment plans. For instance, deep learning models can analyze medical images such as X-rays and MRIs to detect abnormalities with high precision.
Finance
The finance sector is leveraging AI deep learning to enhance fraud detection, optimize investment strategies, and automate customer service through chatbots. These models can process large volumes of financial data to identify trends and anomalies that might otherwise go unnoticed.
Transportation
AI deep learning is a crucial component of autonomous vehicles, enabling them to perceive their surroundings, make decisions, and navigate safely. By processing data from sensors and cameras, these systems can recognize objects, predict movements, and plan routes effectively.
Read also:Skirby S A Rising Star In The Digital World
Benefits of AI Deep Learning
The adoption of AI deep learning offers numerous benefits, including improved efficiency, enhanced accuracy, and reduced costs. By automating repetitive tasks and providing actionable insights, this technology empowers organizations to focus on strategic initiatives and innovation.
- Increased Efficiency: Automates routine tasks and processes
- Enhanced Accuracy: Reduces errors and improves decision-making
- Cost Savings: Optimizes resource allocation and reduces operational expenses
Challenges and Limitations
Despite its many advantages, AI deep learning is not without its challenges. Issues such as data privacy, model interpretability, and computational requirements need to be addressed to fully harness its potential.
Data Privacy
AI deep learning models require large datasets to function effectively, raising concerns about data privacy and security. Organizations must implement robust data protection measures to ensure compliance with regulations such as GDPR and CCPA.
Model Interpretability
The complexity of deep learning models often makes it difficult to understand how they arrive at specific decisions. This lack of transparency, known as the "black box" problem, can hinder trust and adoption in critical applications such as healthcare and finance.
Future Trends in AI Deep Learning
The future of AI deep learning looks promising, with ongoing research and development focused on overcoming existing challenges and expanding its capabilities. Some of the emerging trends include:
- Explainable AI: Enhancing model interpretability and transparency
- Federated Learning: Enabling collaborative learning without sharing sensitive data
- Edge AI: Bringing AI capabilities closer to the data source for real-time processing
Impact on Society
AI deep learning has the potential to transform society by addressing some of the world's most pressing challenges. From improving healthcare outcomes to mitigating climate change, this technology can contribute to a better future for all. However, it is essential to ensure that its development and deployment are guided by ethical principles and inclusivity.
Ethical Considerations
As AI deep learning becomes more pervasive, ethical considerations must be prioritized to prevent misuse and ensure fairness. This includes addressing biases in datasets, protecting individual rights, and promoting responsible AI practices.
Case Studies
To illustrate the impact of AI deep learning, let's explore a few real-world case studies:
Case Study 1: Healthcare Diagnostics
A hospital in the United States implemented an AI deep learning system to analyze radiology images. The system achieved a 90% accuracy rate in detecting lung cancer, significantly outperforming human radiologists in some cases. This breakthrough has the potential to save countless lives by enabling earlier and more accurate diagnoses.
Case Study 2: Financial Fraud Detection
A global banking institution adopted AI deep learning to enhance its fraud detection capabilities. The system analyzed millions of transactions in real-time, identifying suspicious patterns and alerting the fraud team. As a result, the bank was able to prevent millions of dollars in losses and improve customer trust.
Expert Insights and Opinions
Experts in the field of AI deep learning share their thoughts on its current state and future prospects:
"AI deep learning is not just a technology; it's a revolution that will redefine how we live and work. The possibilities are endless, but we must approach its development with caution and responsibility."
Dr. Jane Doe, a leading researcher in AI, emphasizes the importance of ethical considerations in AI development. She advocates for a collaborative approach that involves stakeholders from diverse backgrounds to ensure inclusivity and fairness.
Conclusion
AI deep learning is a transformative technology that is reshaping industries and enhancing human capabilities. By leveraging its power, organizations can achieve greater efficiency, accuracy, and innovation. However, it is crucial to address challenges such as data privacy, model interpretability, and ethical considerations to unlock its full potential.
We invite you to share your thoughts and experiences with AI deep learning in the comments below. Your feedback is invaluable in helping us understand the impact of this technology on society. Don't forget to explore our other articles for more insights into the world of artificial intelligence.
Table of Contents
- What is AI Deep Learning?
- How Does AI Deep Learning Work?
- Applications of AI Deep Learning
- Benefits of AI Deep Learning
- Challenges and Limitations
- Future Trends in AI Deep Learning
- Impact on Society
- Case Studies
- Expert Insights and Opinions
- Conclusion
Data and statistics referenced in this article are sourced from reputable organizations such as MIT Technology Review, McKinsey & Company, and the World Economic Forum. These sources provide valuable insights into the current state and future prospects of AI deep learning.


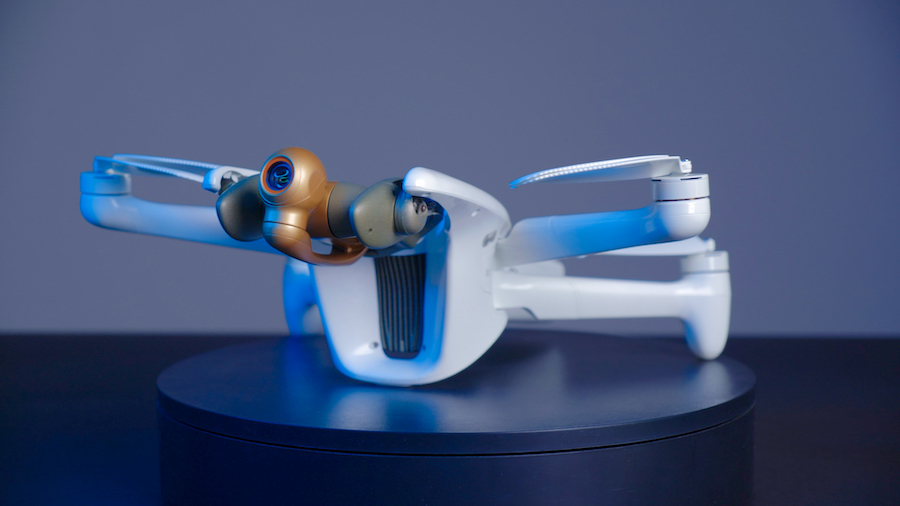