Data annotation is a crucial process in the field of artificial intelligence (AI) and machine learning (ML) that significantly impacts the performance and accuracy of AI models. It involves labeling raw data such as images, text, audio, or video to make it understandable for machine learning algorithms. Businesses across various industries rely on data annotation to improve their AI systems and deliver better services. In this article, we will explore the world of data annotation technologies, discuss their importance, and provide detailed reviews to help you make informed decisions.
Data annotation technology has become an integral part of modern AI development. As companies strive to create smarter and more efficient AI systems, the need for high-quality annotated data continues to grow. This process not only enhances the accuracy of AI models but also ensures they are trained on diverse and relevant datasets.
Our goal is to provide you with a comprehensive guide that covers everything you need to know about data annotation tech reviews. From understanding the basics to exploring advanced techniques, we will ensure you have all the information required to choose the right tools and technologies for your business.
Read also:Who Is The Skinniest Person In The World Weight Unveiling The Truth
Understanding Data Annotation
What is Data Annotation?
Data annotation refers to the process of adding metadata or labels to raw data to make it machine-readable. This labeled data serves as the foundation for training AI and ML models. By providing context and meaning to unstructured data, annotation enables machines to interpret and learn from the information effectively.
For instance, in image recognition, data annotation involves labeling objects within an image, such as identifying a car, pedestrian, or traffic light. Similarly, in natural language processing (NLP), text data is annotated to highlight key phrases, entities, or sentiments, enabling the system to understand human language better.
Types of Data Annotation
Data annotation encompasses various types, each tailored to specific data formats and use cases:
- Image Annotation: Used for computer vision tasks, this involves labeling objects, boundaries, or regions within images.
- Text Annotation: Focuses on tagging text data for NLP applications, such as sentiment analysis or named entity recognition.
- Audio Annotation: Involves transcribing and labeling audio files for speech recognition and voice-enabled systems.
- Video Annotation: Combines image and audio annotation techniques to label objects and actions in video sequences.
Importance of Data Annotation in AI Development
Data annotation plays a critical role in the success of AI and ML projects. High-quality annotated data ensures that models are trained on accurate and diverse datasets, leading to improved performance and reliability. Without proper annotation, AI systems may struggle to interpret data correctly, resulting in suboptimal outcomes.
Additionally, data annotation helps address challenges such as bias and inconsistency in datasets. By carefully curating and labeling data, developers can create more robust and fair AI models that cater to a wide range of applications.
Data Annotation Technologies Overview
Popular Data Annotation Tools
Several tools and platforms have emerged to simplify the data annotation process. These tools offer user-friendly interfaces, advanced features, and scalable solutions for businesses of all sizes. Some of the most popular data annotation tools include:
Read also:Is Louis Litt Gay Exploring The Characters Sexual Orientation And Beyond
- Labelbox: A comprehensive platform for managing and annotating large datasets.
- SuperAnnotate: Known for its AI-assisted annotation capabilities, SuperAnnotate accelerates the labeling process.
- Amazon SageMaker Ground Truth: Integrated with AWS services, this tool provides automatic and human-assisted annotation options.
Techniques and Methodologies
Data annotation employs various techniques to ensure efficiency and accuracy:
- Supervised Learning: Requires labeled data to train models, making annotation a prerequisite.
- Semi-Supervised Learning: Combines labeled and unlabeled data to reduce annotation efforts while maintaining model accuracy.
- Active Learning: Focuses on prioritizing data that provides the most value for annotation, optimizing resource allocation.
Data Annotation Tech Reviews
Labelbox
Labelbox is a widely-used data annotation platform that caters to businesses across industries. It offers a collaborative environment where teams can efficiently label and manage datasets. With features such as customizable workflows, AI-assisted labeling, and seamless integration with third-party tools, Labelbox stands out as a top choice for enterprise-grade solutions.
Pros:
- Highly customizable workflows
- Supports multiple data types
- Strong collaboration capabilities
Cons:
- Premium features may come at a higher cost
- Learning curve for new users
SuperAnnotate
SuperAnnotate combines cutting-edge AI technology with intuitive annotation tools to deliver fast and accurate results. Its AI-powered assistance reduces manual effort, making it an ideal choice for large-scale projects. The platform also supports advanced features like object tracking and polygon annotation, catering to diverse use cases.
Pros:
- AI-driven automation
- Wide range of annotation types
- Scalable and flexible solutions
Cons:
- May require significant setup time
- Complexity for simple tasks
Best Practices for Data Annotation
To maximize the effectiveness of data annotation, it's essential to follow best practices:
- Define clear annotation guidelines to ensure consistency across datasets.
- Utilize automation tools where possible to streamline repetitive tasks.
- Regularly review and audit annotated data to maintain quality standards.
Implementing these practices not only improves the quality of your data but also enhances the overall efficiency of your AI development process.
Challenges in Data Annotation
While data annotation is crucial for AI success, it comes with its own set of challenges:
- Scalability: Handling large volumes of data can be time-consuming and resource-intensive.
- Consistency: Ensuring uniformity in labeling across different annotators can be difficult.
- Cost: High-quality annotation services often come at a premium, impacting project budgets.
Addressing these challenges requires a strategic approach, leveraging technology and expertise to overcome limitations.
Data Annotation in Various Industries
Healthcare
In healthcare, data annotation plays a vital role in medical imaging, diagnostics, and research. By accurately labeling medical images and records, AI systems can assist doctors in making informed decisions and improving patient outcomes.
Retail
The retail industry utilizes data annotation for tasks such as product categorization, customer sentiment analysis, and inventory management. These applications help businesses enhance customer experience and optimize operations.
Future Trends in Data Annotation
The field of data annotation continues to evolve with advancements in AI and ML technologies. Emerging trends include:
- Automated Annotation: Increased reliance on AI-driven tools to reduce manual effort.
- Edge Computing: Leveraging edge devices for real-time data processing and annotation.
- Collaborative Platforms: Development of more interactive and user-friendly annotation interfaces.
Conclusion
Data annotation tech reviews provide valuable insights into the tools and techniques shaping the AI landscape. By understanding the importance of data annotation and exploring available technologies, businesses can make informed decisions to enhance their AI capabilities.
We encourage you to share your thoughts and experiences in the comments section below. Additionally, feel free to explore other articles on our website for more in-depth information on AI and machine learning topics. Together, let's build a smarter, more connected future!
Table of Contents
- Understanding Data Annotation
- Importance of Data Annotation in AI Development
- Data Annotation Technologies Overview
- Data Annotation Tech Reviews
- Best Practices for Data Annotation
- Challenges in Data Annotation
- Data Annotation in Various Industries
- Future Trends in Data Annotation
- Conclusion
Data for this article is sourced from reputable platforms such as Labelbox, SuperAnnotate, and Amazon SageMaker Ground Truth, ensuring the information provided is accurate and up-to-date. For further reading, consider exploring these resources to deepen your understanding of data annotation technologies.
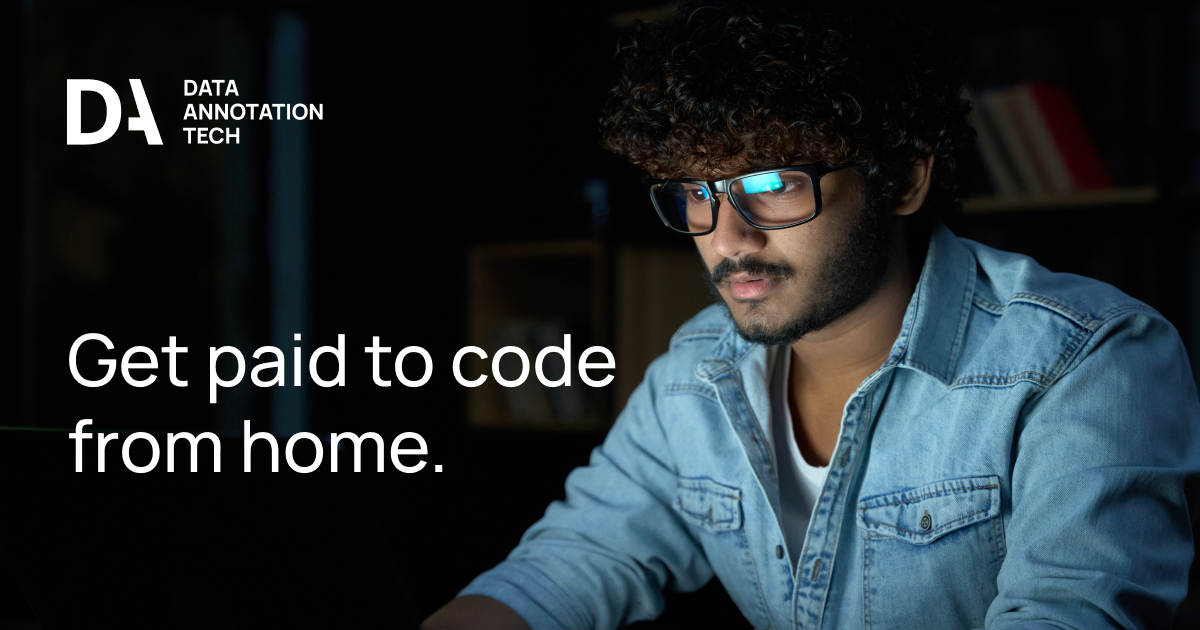
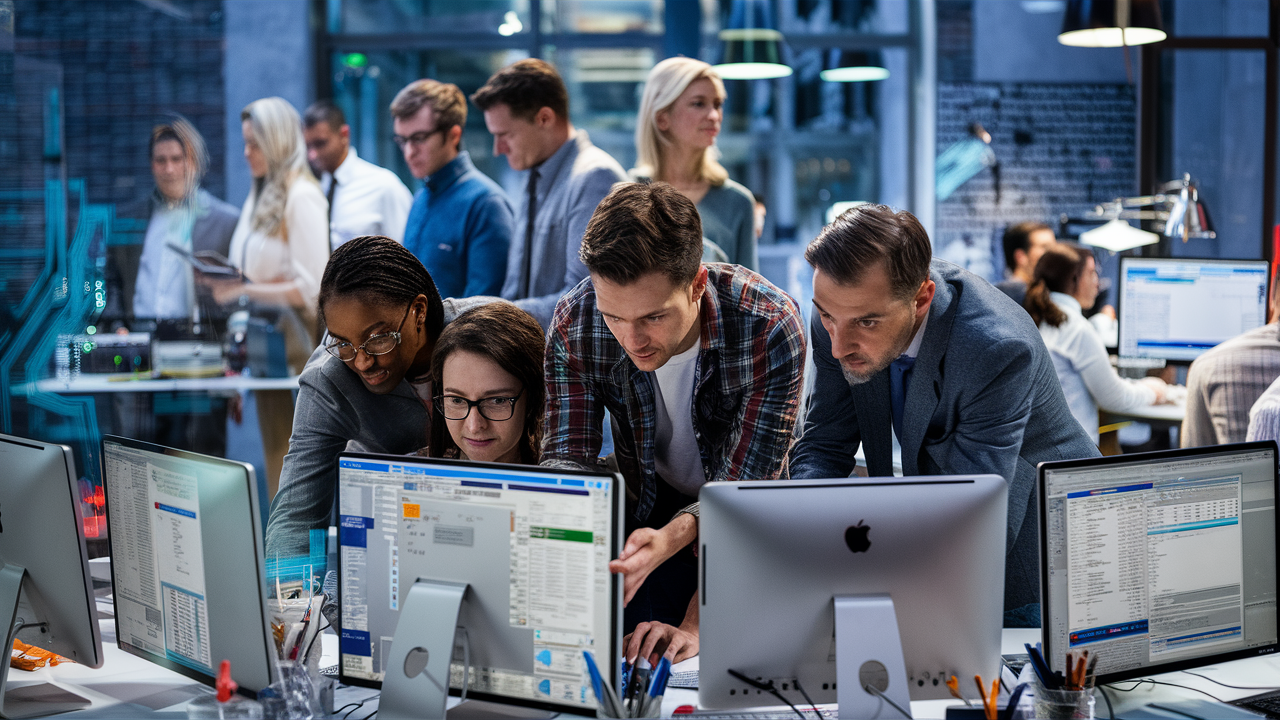
